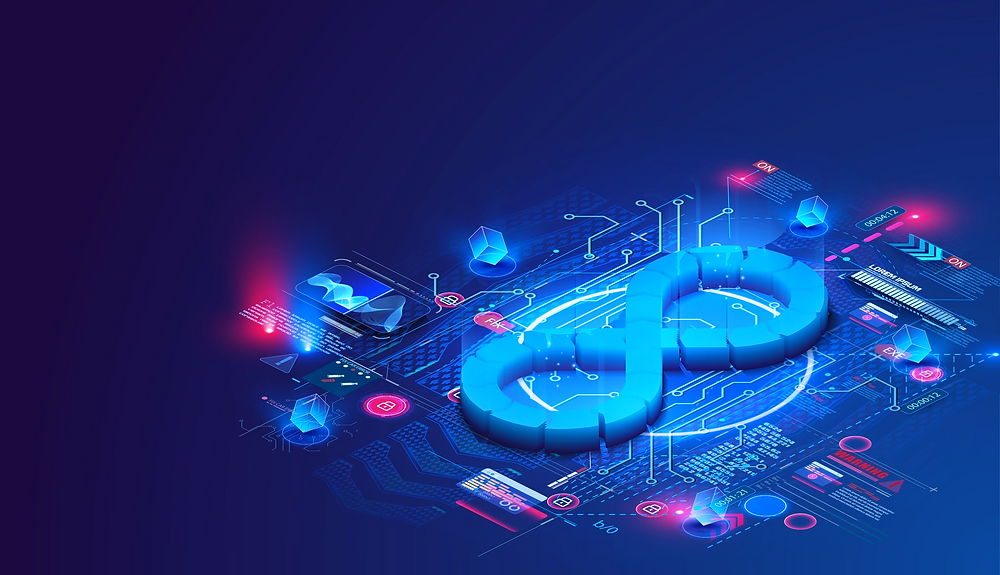
Maximizing ROI: How MLOps Optimizes Business Value from AI Initiatives
In today’s competitive business environment, AI is increasingly popular, offering organizations numerous benefits. However, the introduction of AI into business practice brings challenges related to the management and scaling of machine learning models. That is why the concept of MLOps was introduced. According to the report, the global MLOps market was valued at $983.6 million in 2021 and is projected to reach $23.1 billion by 2023. This just shows how important MLOps is. In this article, we will focus on how MLOps can optimize business value and maximize ROI.
How MLOps are maximizing RoI.
As we know, MLOps are processes, tools, and practices to successfully deploy, manage, and maintain ML models in production. Their goal is to improve performance, quality, and reliability.
According to research, 85% of AI and ML projects fail with no return on business. However, there is a solution that can significantly improve these results – MLOps. MLOps is an approach that provides a more reliable and cost-effective development framework for AI projects. Hence, it accelerates the delivery of successful solutions.
The implementation of MLOps helps organizations to move to larger production environments. But it is important to take into account the fact that AI models must undergo constant change. Once an ML model is deployed, it won’t work the same way for a long time. Model monitoring and testing are essential to ensure that they deliver accurate information.
MLOps helps data scientists to innovate faster when models need to be updated. For example, in the event of a global pandemic or supply chain crisis, it allows you to take appropriate action faster.
Let’s see how MLOps are maximizing RoI.
QUICK DEPLOYMENT OF MODELS
MLOps helps automate the processes of deploying machine learning models. In this way, the time needed to implement the model is reduced, eliminating manual and time-consuming activities. Faster deployment means the company can start benefiting from AI models faster and generate business value. They can reap the benefits, both in terms of revenue growth and cost savings. So, the sooner the model is deployed and available to users, the sooner the organization can start using its potential.
EFFICIENCY SCALING
MLOps contributes to scaling efficiency, which in turn maximizes ROI. Companies can process data efficiently and achieve faster results. Here are some aspects of efficiency scaling.
Automation of operational processes and effective model management – both enable optimal use of resources and focus on generating business value. The use of specialized MLOps platforms ensures the following:
- Central management of models
- Metric tracking
- Performance monitoring
- Easier process of deploying and updating models
With them, organizations have full control over their models. And it translates into greater operational efficiency and greater business value generation. Scaling in MLOps delivers better performance, more accurate results, and greater business value. All of this contributes to a greater ROI for the organization.
MONITORING AND MANAGING MODELS IN PRODUCTION
Monitoring and managing models in production is a key element of MLOps. It allows you to:
- Maintain high-quality prediction and system continuity
- Maximize return on investment
By real-time model performance monitoring, ongoing error diagnostics, and model version management, companies can:
- Quickly detect issues and take appropriate corrective action
- Achieve better financial results
- Increase operational efficiency
- Optimize costs
- And improve the overall performance of their business operations
So, as we can see, monitoring and managing models in production has a direct impact on the return on investment (ROI).
CONTINUOUS IMPROVEMENT OF MODELS
Continuous improvement of models is a key element of MLOps, having a significant impact on the ROI. To continuously improve the model, companies can use techniques such as:
- Reinforcement learning
- Adaptive machine learning
- Hyperparameter auto-tuning
Improving models allows them to adapt to the changing environment, meet users’ needs better, and also improve prediction. High-quality models contribute to better business results and a higher return on investment.
RISK MANAGEMENT AND SECURITY
Security is an integral part of MLOps, affecting the return on investment (ROI). Protecting your organization’s assets is critical to minimizing financial and reputational risks. Security provides the following benefits:
- Protection against data loss
- Breach of confidentiality of information
- Compromise of models
In addition, maintaining the continuity of the organization’s operation is possible thanks to proper security. This, in turn, helps to avoid downtime, loss of customers, and loss of revenue. Customer trust is also critical to ROI. A high level of security builds that trust by protecting data and meeting privacy expectations. Compliance with data protection laws and other regulations is essential to avoid sanctions that can have a negative impact on ROI.
Conclusion
MLOps plaftorm is a key element in optimizing the business value of AI initiatives and maximizing ROI. Companies can effectively implement and maintain ML models in production by:
- Automating processes
- Monitoring and managing models
- Continuous improvement and
- Paying attention to security
Generally, MLOps enables organizations to use the full potential of AI and thus achieve an impressive ROI.