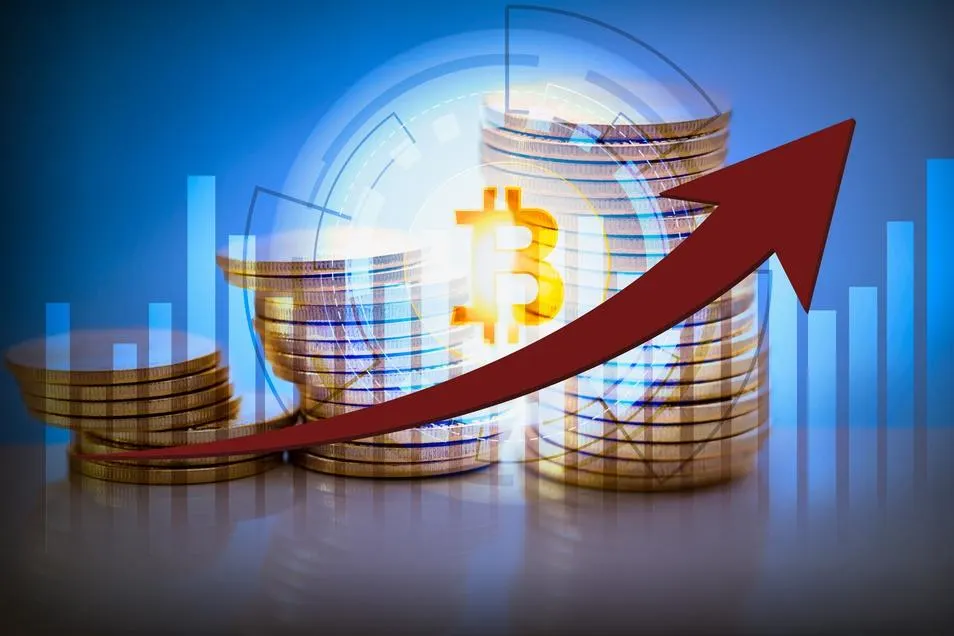
Demystifying Crypto Price Prediction Models: A Comprehensive Guide
Cryptocurrency markets are notoriously unpredictable, often experiencing dramatic price swings within short timeframes. Both traders and investors seek ways to predict these price movements to make informed decisions and maximize profits; one method for doing this is through price prediction models; however understanding and using them effectively may prove challenging due to their complex design, unpredictability of crypto markets, as well as various methodologies involved with them – we will debunk all that complexity while discussing strengths/limitations/practical applications of these models within this comprehensive guide.
Understanding Crypto Price Prediction Models
Crypto price prediction models utilize various techniques, such as statistical analysis, machine learning and artificial intelligence, in order to forecast future price movements of cryptocurrencies. These models sift through historical price data as well as market indicators, trading volume, social media sentiment analysis and other sources that might impact prices in order to identify patterns or trends which might impact prices in order to make predictions on their movements.
Prediction Model Types
Statistical Models:
These predictions use statistical techniques such as linear regression, time series analysis and moving averages to forecast future price movements. These predictions often use techniques such as linear regression analysis or time series analysis in order to spot patterns or extrapolate trends over time.
Machine Learning Models:
Machine learning models rely on algorithms that iteratively learn from data, adapting and improving predictions over time. Such models can analyze vast quantities of information while uncovering intricate patterns not apparent to human analysts read more https://blockchainreporter.net/price-prediction/.
Sentiment Analysis Models:
Sentiment analysis models examine social media posts, news articles and other sources to gauge investor and market sentiment and investor behavior. By understanding collective emotions of market participants these models attempt to predict future price movements by understanding collective sentiment patterns.
Hybrid Models:
Hybrid models combine multiple techniques and data sources in order to improve prediction accuracy. For instance, they may combine statistical analysis and machine learning algorithms in order to capture different aspects of market dynamics.
Before diving in to specific prediction models, it’s essential to gain a solid grasp on all the various influences on crypto prices. While cryptocurrency valuations tend to be driven by speculation alone, several key aspects could potentially change this dynamic:
Market Sentiment:
Investor sentiment plays a pivotal role in crypto markets, shaping buying and selling decisions based on perceptions of future market conditions and potential returns.
Regulatory Developments:
Government announcements and actions by regulatory bodies can have a substantial effect on crypto prices, impacting investor trust and market dynamics.
Technological Developments and Inventions in Crypto:
Technological advancements within the cryptocurrency space such as upgrades to blockchain protocols or the inclusion of new features can have a direct effect on investor sentiment and market demand.
Market Liquidity:
Liquidity refers to how easily an asset can be bought or sold without significantly impacting its price, such as cryptocurrency trading pairs with higher liquidity experiencing less price volatility compared to those with lesser liquidity.
Market Manipulation:
Due to their relative unregulation, crypto markets can be vulnerable to manipulation from individuals or groups looking to influence prices for personal gain.
Popular Prediction Models and Techniques
Moving Averages Moving averages are an effective yet simple price prediction technique used in price prediction models. By calculating an asset’s average price over an extended time span (say 10 days or 50 days), moving averages help smooth out price fluctuations, providing smooth price increases over time and potentially providing entry and exit points in trading transactions. Moving averages have also proven helpful to traders by helping identify trends as well as entry or exit points during trading activities.
Relative Strength Index (RSI)
The Relative Strength Index (RSI) is a momentum oscillator which measures price movements at regular intervals and tracks them over time. Ranging between 0 and 100, traders use it to detect overbought or oversold conditions in the market and can interpret RSI readings to predict whether an asset’s price reversal or continuation might occur in response to certain triggers in an RSI reading.
Support and Resistance Levels
Cryptocurrencies typically find support or resistance at certain price levels that provide buying or selling pressure, often identified based on historical price data and used as trading indicators by traders to anticipate price movements. When cryptocurrency breaks above a resistance or support level it could signal either trend reversal or continuation and traders should remain wary when these levels break unexpectedly.
Machine Learning Algorithms
Machine learning algorithms such as neural networks, decision trees and support vector machines have become an indispensable part of developing accurate crypto price prediction models. These sophisticated models analyze vast amounts of historical data in search of patterns not easily discernible with traditional analysis approaches.
Sentiment Analysis
Sentiment analysis models utilize social media posts, news articles and other sources of data to measure market sentiment and investor behavior. By understanding collective market participant emotions and anticipating how future price movements might respond accordingly
Limitations and Challenges
Although crypto price prediction models provide valuable insights, they also face certain restrictions and challenges:
Market Volatility:
Cryptocurrency markets are highly unpredictable, making accurate forecasting difficult even with advanced models.
Data Quality:
The quality and reliability of the information used to train prediction models can have an enormous effect on their accuracy and efficacy.
Changed Market Conditions:
Crypto markets can be subject to many influences and the conditions may rapidly shift, making it hard for models to adapt quickly enough and produce accurate predictions.
Overfitting:
Overfitting occurs when a model incorrectly learns to fit noise in its data rather than identify patterns beneath. Overfitted models may perform well on historical information but cannot generalize to new data sets.
Lack of Transparency:
Some prediction models that utilize machine learning techniques can lack transparency, making it hard for us to comprehend their predictions and how they were made.
Practical Applications
Crypto price prediction models do have some practical applications:
Risk Management: By anticipating price movements, traders and investors can implement risk-management strategies in order to limit losses and protect their investments.
Algorithmic Trading Systems:
Algorithmic trading systems allow traders to more quickly capitalize on market opportunities with automated trade execution based on signals generated from prediction models, making use of algorithms an essential asset in trading operations.
Market Analysis:
Price prediction models can assist analysts and researchers with gathering insight into market trends, investor sentiment analysis and emerging patterns within the crypto ecosystem.
Portfolio Optimization:
Investors can utilize price predictions to craft more balanced investment portfolios by allocating risk and return across various assets or asset classes.
Conclusion
Crypto price prediction models play an essential part in helping traders and investors navigate the volatile and dynamic cryptocurrency markets. Utilizing statistical, machine learning and sentiment analysis techniques, these models attempt to predict future price movements and identify trading opportunities. However, it’s essential to recognize the inherent limitations and challenges posed by prediction models when making investment decisions based on them. With cryptocurrency markets continually developing and changing rapidly over time, prediction models will remain an active field of research and innovation shaping the future of cryptocurrency trading and investment strategies.