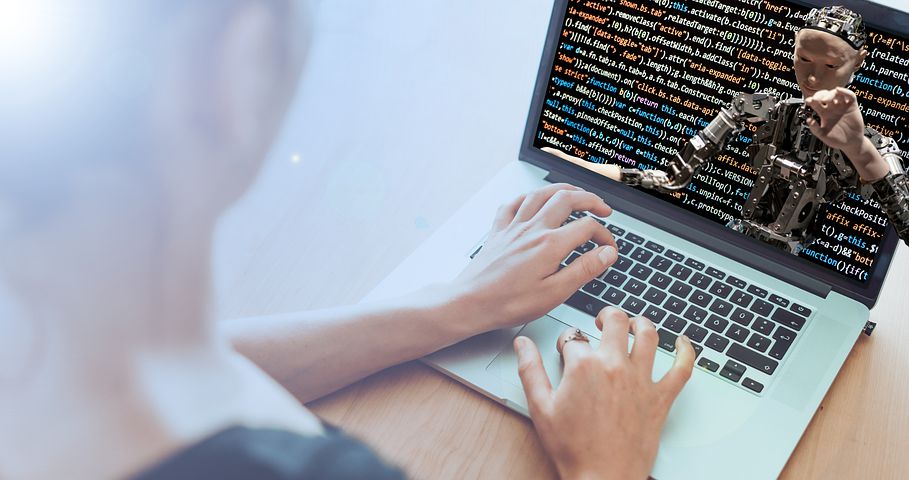
How Is AI Unlocking New Possibilities For QA?
The use of AI in quality assurance (QA) is a silent revolution that is assisting industries like automotive in resolving hardware constraints and test cycle bloat. Artificial intelligence’s greatest effective reception in QA is examining code changes to run just basic tests, talks about co-Chief and pioneer behind Launchable, Harpreet Singh.
The software development life cycle (SDLC) has served as a seedbed for incorporating AI into development over the past few years, despite the hype surrounding AI chatbots and even AI in crypto. Various QA blogs talk about this.
The software testing industry is now ready for advancement thanks to the maturation of CI/CD (continuous integration and continuous delivery). The end-users demand for quality and faster throughput drives the adoption of AI in software testing, despite the general buzz surrounding AI.
SDLC procedures are only getting more complicated. The only way for teams to combat complexity and shorten test cycles is through data-driven pipelines. While the new rush of QA testing and artificial intelligence came in like a sheep, the reception of simulated intelligence in programming testing is spreading and gradually feeling more like a lion.
Although testing continues to be a significant source of friction in the majority of release cycles, it is still the phase of the software development life cycle that is given the lowest priority in terms of the advancement of the technology stack. Due to large test suites, problematic tests, and a general reliance on manual testing, software testing frequently becomes the obstacle preventing developers from achieving faster and more reliable release cycles.
Artificial intelligence is having the greatest impact on testing. By its temperament, artificial intelligence can add esteem by using the information to mechanize monotonous cycles. The tsunami of data generated by software testing is being ignored. Utilizing this information wisely can lessen the bulge felt by design groups. Incorporating AI-assisted testing, designer erosion can be significantly diminished, prompting further developed engineer insight and work environment effectiveness.
To improve software testing procedures, developers and quality assurance teams frequently adopt AI in two scenarios: having either too many or too few tests.
Having an adequate number of tests is fundamental for quickly and precisely recognizing blunders. If there aren’t enough tests, developers run the risk of finding bugs before moving on. Thus, botches compound and are more challenging to address. Man-made intelligence test-creating instruments like Mesmer have acquired prevalence, permitting groups to foster all the more great tests for their testing program. This has been discussed in numerous QA blogs.
In contrast, businesses frequently encounter testing pipelines that are bloated due to an excessive number of tests. This makes testing a basic bottleneck and risk. 75% of DevOps professionals polled in 2022Opens a new window said that testing cost them more than 25% of their productivity, indicating that over-testing is still hurting team throughput.
It can take a long time and run the risk of producing false positives and negatives to run unnecessary tests for each commit. As a solution to this issue, some teams are utilizing AI to make large-scale testing possible. Utilizing machine learning models to gather test suite insights and optimize test selection can be examples of this.
Predictive test selection is the next-generation artificial intelligence approach to its manual counterpart, test impact analysis. There are numerous methods for evaluating the impact of code changes on tests. Using a machine learning model that has been trained on previous test results, it chooses which tests to run for a given code change. Teams are allowed to analyze every project using AI, allowing for faster testing cycles and enhanced code confidence. The increasing use of AI in software testing is accelerating release cycles and allowing developers to concentrate more closely on innovation.